The insurance industry is in an exciting place right now. It is a calm time before the storm. There are multiple changes in the world, especially when it comes to artificial intelligence, which brings earthquakes.
The traditional approach of selling protective products is nowhere near enough for the insurer of the future. Digital disruption and demanding customers mean that insurers need to innovate to stay relevant within their industry.
The pace of turbulence has speeded up recently. Spooked by the effect that artificial intelligence has had on industries from healthcare and retail to banking and eCommerce, insurers wonder if they are next in line for disruption.
Insurtech reshapes world with Artificial Intelligence and more
According to Deloitte, approximately $2.6bn was invested in “insurtech” businesses in 2018, and a considerable part of the money funded AI in Insurance. The amount jumped to $6.37 billion, according to data from Willis Towers Watson.
Insurers are also reacting to changing expectations from customers who face new risks and want to know how the insurance industry can uniquely help them.
There are a few strong forces changing insurance landscape, all of them based on Artificial Intelligence applications or AI-driven approach:
- Telematics and Usage-Based Insurance, which move the needle toward highly personalized offerings. The classic example is personalized auto insurance. Those who drive very little pay different rates than those who drive a lot. Smart devices made it possible to charge people based on the miles they drive.
- The Internet of Things (IoT) is the new era where many items, especially appliances, will be equipped with tiny processors allowing us to take full advantage of whatever data they collect. The risk management possibilities will be amazing with the support of Artificial Intelligence algorithms. We can easily imagine being able to react immediately to claims and prevent claims entirely by detecting ahead of time that your oven is in danger of causing a fire.
- Blockchain, by bringing decentralization for money transfer and smart contracting, affects claims payments, claims processing, and contract signing.
- Bots that step in when the lengthy form, complicated questionnaires, sophisticated background check, and robust claims settlements make customers unhappy. AI-fueled bots have ushered in new standards by embracing video, text, and voice recognition.
- Ownership redesign happened, and a large part of society doesn’t want to own things. Instead, they prefer to rent, share, or hire. AI in the insurance industry helps to find new ways of risk and fees calculation and predict the future of items. The shared car is getting old differently than the one who is owned.
How can AI help the insurance industry?
As digital channels become increasingly relevant for connecting with customers, insurers in pursuit of high performance need to develop a customer-centric and digital mindset, making critical changes to their operational processes and becoming a genuinely customer-focused enterprise.
The very first touchpoint, which builds the first impression and can either convince costumers to stay with the company or move to competitors, is the claims cycle. After checking the insurance price, most customers are curious how much time it takes to settle the claim, which states for critical decision-making points.
Claims life cycle in insurance starts with filing an insurance claim which usually is the most pleasant experience that you’ve likely ever encountered. Stress, unclear process, a lot of manual tasks to handle are one of the most unpleasant components of the customer journey.
Artificial Intelligence doesn’t have emotions
AI can act fast and automate, plus it can predict a lot as well.
Let’s see how can Artificial Intelligence in the insurance industry changes the claims settlement process.
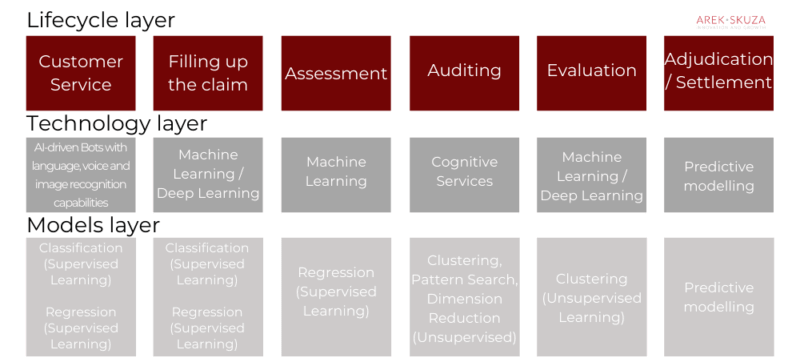
While a new era of claims settlement standards is being set with AI, the industry is turning its attitude towards embracing the real potential of the machine and deep learning that can save valuable time and money from the firm’s bottom line.
For this entire flow to materialize — from the customer “Filling up the claim” to receiving the amount in a bank account within a short amount of time, a set of different supervised and unsupervised algorithms and models is required. Deep Learning is helpful too, especially in image and photo processing.
AI technologies make processes more transparent, automated and improve the interaction between customers and the insurance company. By doing this, AI gives insurers an edge on how they manage claims—more agile, more reliable, and with fewer errors.
What insurtech does differently and the insurance industry needs to adopt?
Insurers can achieve better claims management by using intelligent technologies in some of the following ways (referring to the picture above):
- To enable a real-time question-and-answer service for first notice of loss (Customer Service)
- To pre-assess claims and for automated damage evaluation (Filling up the claim and Assessment Layer)
- To enable computerized claims fraud detection using enriched data analytics (Auditing and Evaluation)
- To predict claim volume patterns (Adjudication / Settlement)
[elementor-template id=”3798″]
Artificial Intelligence speeds up insurance
We have conducted a pilot and study with one of the insurance companies on the application of AI to their home insurance claims processes. This insurer’s health claims management process took from 5 – 10 minutes from the claim registration to the final decision.
Figure 1 presents the set of algorithms and methods the company leveraged to speed up the claims journey.
Artificial Intelligence-driven bots
According to IBM, Chatbots can help businesses save 30% on customer service costs by shorter response times and responding up to 80% of routine inquiries. Bots automate most of the process explained in Figure 1. The insurance industry chooses Artificial Intelligence-driven bots more often, as they boost productivity up to 80%.
From streamlining the complete customer service process to instant access, chatbots are proving a hit with customers – especially if the customer journey is personalized, more transparent, and saves time. The most appreciated features by the customer, in our case study, are:
- ability to recognize quickly addresses and locations,
- gathering items parameters for further offer preparation
- understanding the context of circumstances
- recognizing videos that can deliver various information
- emojis and other short forms, which speed up the conversation and make it mobile-friendly
- recognition of items and real estate pictures instead of expecting typing or phone calls.
Applying Image Recognition to Insurance Industry
How can the insurance industry leverage photo recognition technology?
The first reason is that images provide new and useful information that conventional data sources cannot deliver.
For instance, if we see a picture of a motorcyclist using a cell phone while riding, it tells us that the biker is more likely to be a high-risk customer. Insurance powered by machine learning can spot risky points.
The second reason is that image recognition can speed up specific tasks and reduce waiting time. Instead of uploading PDF and wait for a person to read it, character recognition technology can extract data on the fly and match it with an existing profile. It can also check person photos for updating, which is vital in claim processing.
Why Machine Learning is unstoppable
But why should we rely on a machine learning-powered system instead of the human eye? From a resource aspect, the photos database might be too bulky for a team to process efficiently and timely. From a cost view, it may be cheaper to use machine learning models than humans to squeeze information from images.
Image recognition techniques are already widespread in many industries, and in our case the most effective are the followings:
- Identify users and people authentication,
- automatic photo tagging,
- searching for objects within the video,
- product image matching.
Mentioned techniques are widely used in social media services, online shopping, and mobile services. The information builds insurers’ profiles, which companies can use in assessing the insuring risk.
Other applications of AI-driven image recognition in the insurance industry
Some other applications in the insurance industry are listed here:
1. Fraud detection. Facial recognition can reduce the fraudulent use of data and insurance services. For example, facial recognition may be required for an online customer for authentication purposes.
2. Verification of signatures or handwriting. By automatically evaluating the association of a signature to the one on record, a customer’s identity can be verified to approve claims and transactions. For example, a form can be deposited by taking a photo of it.
4. Personalized service. Facial biometrics can be used to identify VIP clients and provide customized services to improve customer satisfaction. Personalization is a huge topic in the insurance industry, as it can bring more margin and increase customers satisfaction.
5. Interactive marketing. Based on potential customers’ image data on social networks, marketing and sales efforts can be tailored for better success. Customers may be classified into different types with corresponding marketing strategy and product solutions.
6. Health insurance is another area where image recognition can provide more insight regarding the risk of individual customers. Physicians can use image recognition for diagnosis and prognosis. Medical images such as computed tomography scans of cancer may be used directly as pricing factors. Scans are used to discover whether a patient has lung cancer, how severe it is, and how it affects the insurance price and future medical treatment.
Combating Insurance Fraud With Machine Learning
Machine learning can allow insurers to shift from the current state of “spot and react” to “predict and prevent.” It shines at automating the process of using high volumes of data, examining various fraud indicators in parallel, and finding potential fraud.
Generally, there are two methods in fraud detection to teach or train an Artificial Intelligence algorithm, which depends on the accessible data: One is supervised and the second is unsupervised learning.
Supervised and unsupervised models in the insurance industry
In predictive modeling or supervised learning, algorithms make predictions based on a set of samples from historical data. A company can feed an algorithm with historical insurance claims and associated results called labeled data.
It will try to recognize the underlying patterns in fraudulent claims. Once the algorithm has been trained on past cases, a business can use it to deduce the chance of a new claim being fraudulent.
A great example is AKSigorta Insurance which leveraged predictive modeling as part of its claims investigation process. The company increased its fraud discovery rate by 66% and is now capable of preventing fraud in real-time.
There is a wide variety of predictive modeling algorithms to choose from, so users should consider issues such as accuracy, interpretability, training time, and ease of use. There is no single approach that works universally. Even experienced data scientists have to try different methods to find the right algorithm for a specific problem.
It is, therefore, best to start simple and explore more advanced machine learning methodologies later.
Decision trees, for example, are an excellent way to start exploring complex relationships within data. They are relatively easy to implement and fast to train on large volumes of data. More importantly, they are very easy to understand or interpret, and can be a good starting point for new business rules.
Related reading: Artificial Intelligence and product management what managers need to learn.
Unsupervised algorithms are instructed against data with no past labels. The algorithm is not provided with the answer or expected result beforehand. It is simply asked to investigate the data and reveal any “promising” patterns.
For example, given certain behavioral information, unsupervised algorithms can recognize clusters and mini-clusters of customer transactions that look alike. Anything that appears different or unique could be flagged as an anomaly and sent for further study.
Unsupervised learning methods can, therefore, recognize both existing and new kinds of fraud. They are not limited to predefined labels, so they can promptly adapt to new and emerging patterns of dishonest behavior. For example, an Asian bank used unsupervised learning methods to identify cases where agents were overcharging customers for a particular procedure or upselling unnecessary products, which influence churn negatively.
Unsupervised detection methods include:
- univariate outlier analysis,
- clustering-based methods such as k-means.
Brand-new algorithms, such as Support Vector Data Description or Isolation Forest, have been effective in detecting anomalies and allowing faster reactions to new types of fraud.
Claims Adjudication and unsupervised learning
Claims adjudication refers to a process in which the insurance company reviews a claim it has received and either settles or denies it after due analysis and comparisons with the benefit and coverage requirements.
The process begins with receiving the claim, either directly from the policyholder or the provider.
Once the process of adjudication is complete, the insurance company makes the payment and/or sends a letter to the claimant, describing the company’s decision to accept or reject the claim, along with validating reasons for doing so, and how the amount to be paid was determined. This is known as the remittance advice or, more popularly, Explanation of Benefits (EoB).
The letter also includes, for an accepted claim, detailed information about how each service included in the claim was settled and how much is being paid.
Problems Faced in Claims Adjudication
The claims adjudication process is riddled with challenges because:
- Claims are submitted through a variety of mediums – electronic and manual,
- a dedicated workforce is required to manually verify each claim, filter, and investigate suspicious claims through a long and cumbersome process,
- manual rule-driven mechanisms are time-consuming and pose threats like loss of customer base due to delays or perceived harassment over submitted claims,
- erroneous rejection of genuine claims or delayed settlement of claims can cause customer dissatisfaction,
- several billions of dollars are lost due to fraudulent claims each year, missed out by inefficient adjudication process.
The significant elements which machine learning supervised and unsupervised algorithms can help with:
- Conversion of claim data from existing PDF formats (patient details, diagnosis details, diagnosis tests, treatment details, and bill summary) into structured formats,
- points out to anomalous elements within claims (bills, procedure),
- payment determination is made easy through Ai-driven software that uses big data analysis and supervised / unsupervised learning to estimate the average and reasonable amount usually charged for specific services,
- the automation of the claims adjudication using Artificial Intelligence makes the entire process of claims adjudication easy, effective, and transparent. More claims can be processed in less time, as AI-fueled software can scale faster than traditional technologies.
Artificial Intelligence in the insurance industry – case studies
What would be agitation without evidence?
Please find these two case studies, which explains how Artificial Intelligence-driven technologies are reshaping the insurance industry.
Case 1: Allstate – artificial intelligence application in agents workload management
Allstate partnered with a consulting company to develop a virtual assistant.
It is fascinating Artificial Intelligence in the insurance application.
The bot, named ABIE (Allstate Business Insurance Expert), was built to help Allstate agents seeking information on Allstate B2B insurance products.
Before the bot was launched, agents mostly sell Allstate line products such as health or homeowners insurance. When the insurer changed the business strategy and started selling commercial insurance, numerous agents had a steep learning curve. They faced challenges accessing the information they needed to communicate with potential customers effectively.
As a result, Allstate found their pre-sales support call center consistently flooded with requests from agents and ultimately experienced rasing churn and growing pool of lost business opportunities.
A virtual assistant is an excellent example of an Artificial Intelligence application in insurance. The bot, who appears as an avatar, reportedly provides agents with step-by-step guidance for building commercial proposals using natural language. ABIE processes 25,000 inquiries monthly, without human touch.
Case 2: Sunday – application of Artificial Intelligence in creating a premium offering
Sunday, which is based in Thailand, is a leading InsurTech company that leverages AI-powered solutions to increase conventional insurance services’ efficiency.
For instance, Sunday uses Machine Learning algorithms to provide customers with:
- a more comprehensive range of insurance products, covering properties,
- highly customized premiums that deliver a more compelling value proposition to customers.
Sunday is enabling customers to purchase insurance policies that reflect the underlying risks more precisely. For instance, leveraging Unsupervised algorithms, the company answered the market demand with precision and speed. Customers were expecting more flexible car insurance policies. By analyzing a vast amount of data, Sunday created Weekend Car offering, where customers don’t pay for the days when the vehicle is not in use.
Since launching in August 2017, the company’s revenue has been growing by 30 percent month-on-month as the new offering attracts more customers thanks to a high level of AI-driven personalization.
Related reading: Why you might never catch up. Grab AI today.