New product development (NPD) is designing and launching products to answer a market opportunity.
AI for new product development has applications across various business sectors. In the automotive vertical, companies consider a combination of smart algorithms to manufacture cars faster, make cars environment friendly, and safer while meeting production cost and volume considerations. In the traveling vertical, the business considers a combination of different experiences to deliver experience, fun, and relax without getting out of the home or country. COVID10 complicated a lot in the traveling business. Airbnb discovered experiences that let people enjoy time together without traveling and renting rooms or apartments.
Time is a factor in new product development, and startups know it
New product development is a process, infamous for how time-consuming it can be, noted by some to sink up to 50% of development time.
Artificial Intelligence (AI) allows for compelling digital testing and predictions of product prototypes before a business dedicates time and resources to physical product experiments. Often, future products vary on a spectrum of traits. Startups know that!
Startups are fast-moving players who understand the need to keep cost regimes and bring results as quickly as possible. Founders have already decided that AI can help them cut time-to-market, which delivers faster product-market-fit stage.
Startups can’t complain, their requests are heard by investors. in 2019, AI-powered products magnetized $16.5B, and the number is growing. Thousands of AI-related job postings are available on the Internet, which is strong evidence that smart algorithms are rocking the product development world. Almost 16 000 job positions for product development engineers are visible on LinkedIn, Monster, and GlassDoor.
Lemonade redesigned claims and insuring process, and gave it to the hands of Machine Learning, bagged first 100 million dollars within a year. Artificial Intelligence is the key and starts small, scale fast paid off.
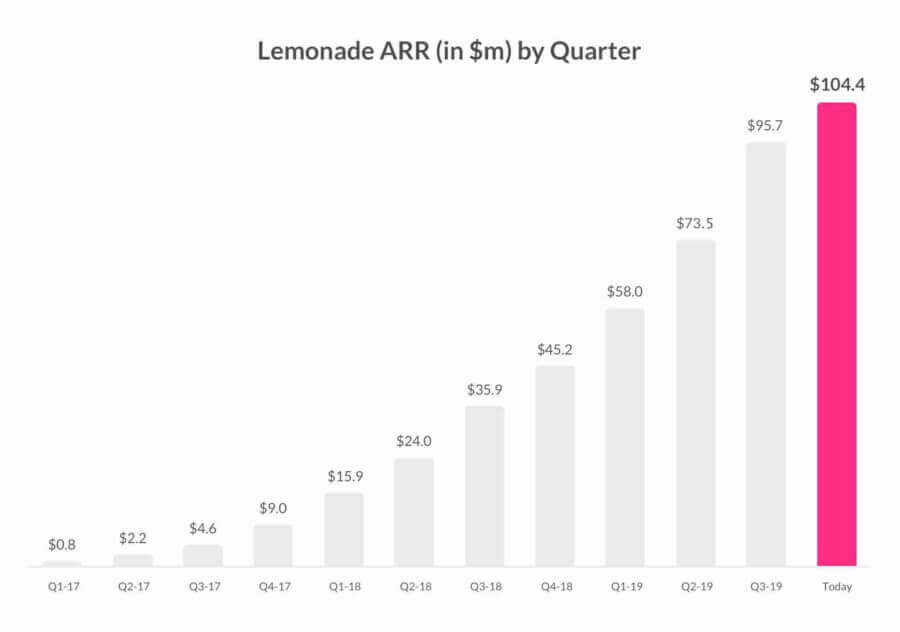
Figure 1: Machine Learning powered Lemonade unbelievable revenue growth
Enterprise leveraged fully integrated New Product Development process
PwC found that digital product development is expected to boost efficiency by almost 20% and reduce product launch time by 17%. Let me combine this with the last achievement of Yelp. The company hired Artificial Intelligence to run front-end tests. Instead of classic A/B testing, Yelp runs almost 700 tests simultaneously. They can find great buttons, headlines, UX structure faster than before. It cuts the time-market by more than 20%.
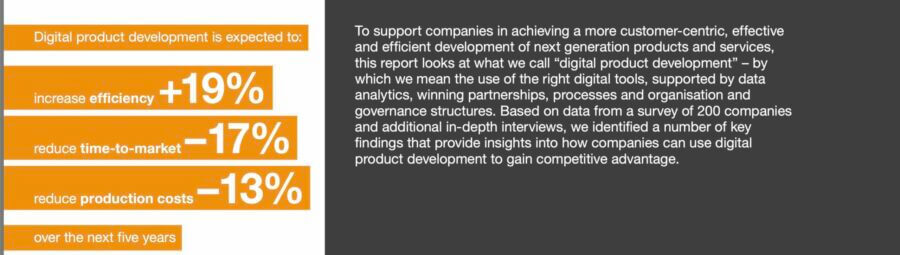
Figure 2: PwC claims companies will launch products faster, thanks to Machine Learning and Deep Learning technologies
Fast and accurate New Product Development needs an integrated partner ecosystem that focuses on co-creation. In other words, great products are built with partners, not in the darkroom. Digital champions understand how to build partnerships that help to deliver fantastic customer experience. The case study of a global logistics company explains that co-creation can reduce a new product launch budget by 60%.
Artificial Intelligence algorithms only get smart when they are fed with high-quality data. Usually, the data stack, which the specific company owns, has flaws. Strategic partnerships are critical in improving data quality, which influences prediction accuracy. Amazon partnered with many clinics in the USA to train Alexa to understand coughing, hawking, and other sounds.
PwC report states that new product development teams, which integrated AI-powered tools into digitalization, achieve higher economies of scale, productivity, and velocity in the product design and development process.
AI as the magic ball in New Product Development
In the research, published in the Journal of the American Medical Informatics Association (JAMIA) Open, the researchers trained AI models to predict food product recalls from Amazon reviews with about 74% accuracy. The AI-powered software then utilized Amazon reviews to recognize thousands of potentially unsafe food products that have not yet been discovered.
AI can predict whether the particular product will be dangerous, not useful, defective, or will not meet the demand. Think about BMW, Boeing, or any food startup. If CIOs applied enough data, these companies could see the future of their products. Afterward, AI gives them the chance to correct a new product development process accordingly.
How to build the AI-powered, future seeing, glass ball? This kind of software harvest social media, websites, search engines and apply algorithms such as natural language processing (NLP), image classification, image recognition, and various deep learning techniques, to get even deeper insights into customers, helping businesses lean not just what customers are looking for and how they are using products today, but also to predict better what features clientele may want in the future.
Remove roadblocks in a New Product Development process
Eliminating the roadblocks to getting new products launched starts with using AI to improve demand forecast accuracy. Honeywell uses AI to reduce energy costs and negative price variance by tracking and analyzing product price elasticity and price sensitivity. Honeywell is integrating AI and machine-learning algorithms into procurement, strategic sourcing, and cost management getting stable returns across the new product development process. (Source: Honeywell Connected Plant: Analytics and Beyond. (23 pp., PDF, no opt-in) 2017 Honeywell User’s Group)
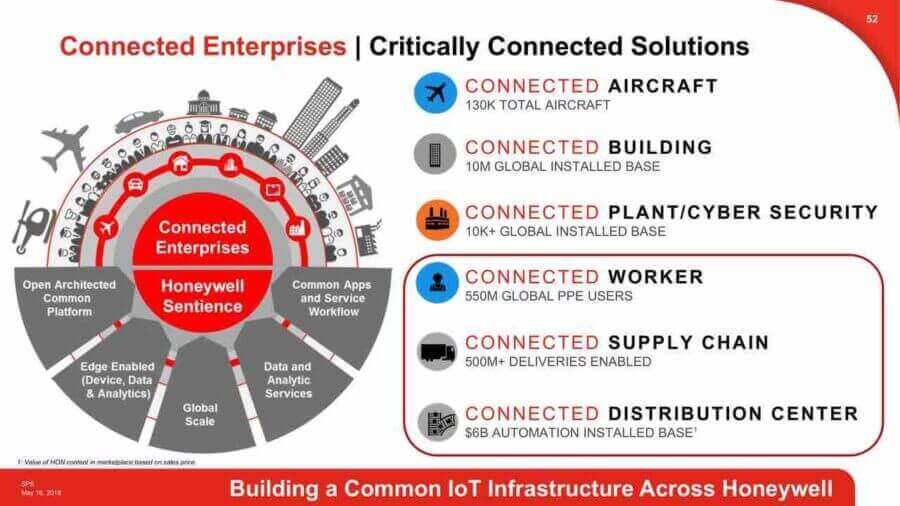
Figure 3: Honeywell connected plants diagram and data business structure
Businesses want to know the future, as they can then reverse engineer to adjust supporting functions. Money management, risk management, supply, and procurement generate huge costs in any business. Honeywell can remove uncertainty by redesigning procurement and sourcing in product development process.
Another excellent example of conducting New Product Development with AI is Analytical Flavor Systems. Who is your biggest problem? It’s your competitor. AFS leverage vast landscape research and build preferences matrix. It helps to understand what drives the market, meaning, map put food and beverages flavors which people love. Afterward, AI-powered engines design flavors that are not offered by competitors, but it is almost certain, people will enjoy those flavors. The company developed an AI-fueled platform, which assists a New Product Development process and can model human sensory perception and predict preferences. Also, the system can read competitors’ offering, which helps the AFS customers, remove the most critical roadblock – a competitor.
AI in New Product Development is a backbone, not an add-on
PWC report explains that digitally mature companies use data analytics powered by AI comprehensively. These companies are applying different AI applications across business functions. It reduces or removes bottlenecks, converts manual tasks to automated experience, and makes data-driven decisions a reality.
Companies that leverage Artificial Intelligence in a New Product Development focus their attention not only on algorithms, programming, and data engineering but also on the underlying data models that make the process possible and efficient.
68% of digitally advanced companies, which PwC surveyed, implement, and integrate AI in product development to optimize Design for Excellence. 80% of production costs are defined during the product development process. If a particular company can plot 3D printing into the process of manufacturing, the Total Cost of Ownership will be lower than when using traditional components.
Also, 91% of companies that leverage AI in NPD don’t want to launch products that nobody wants. This is the primary reason for using smart models in NPD. AI helps to validate products and services before they are officially launched. Startups’ founders know that. They often open subscriptions for the product before the particular app or website exist. Feedback, clicks, and heatmaps help to understand what customers want and feed AI engines, which can then predict the product shape.
If your business wants to leverage AI in a new product development process, you need to consider redesigning the whole product management function and making the AI a flywheel. Otherwise, your AI will be an add on, not a backbone.
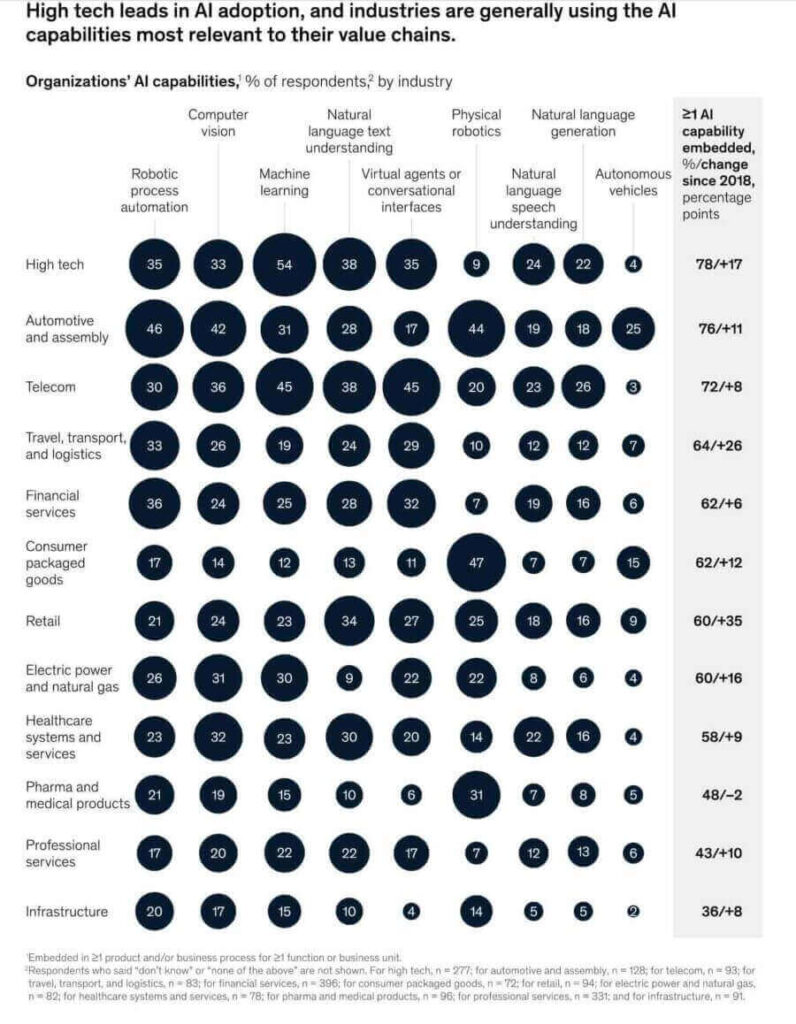
Figure 4: McKinsey research results defining AI adoption in product development
Intelligence AI as the backbone of product management
Uber is a fantastic example of installing Artificial Intelligence, specifically machine learning, in the company DNA. The company created what is called Michelangelo.
Michelangelo empowers internal teams to seamlessly create, deploy, and operate machine learning at Uber’s scale. The platform, which, in fact, is a product itself, is designed to cover the end-to-end machine learning workflow: starting from data acquisition, data evaluation, models training, evaluating results, to monitoring predictions.
Michelangelo has been serving production use cases at Uber for about a year and has become the de-facto system for machine learning for our engineers and data scientists, with dozens of teams building and deploying models. In fact, it is deployed across several Uber data centers, leverages specialized hardware, and serves predictions for the highest loaded online services at the company. – Jeremy Hermann, Head of Machine Learning Platform at Uber
Michaelangelo presence in the product development process
UberEATS has numerous models running on Michelangelo, covering food delivery time predictions, search rankings, and restaurant rankings, are a few examples. Artificial Intelligence powers different parts of the Uber Eats digital products. The delivery time models predict how much time is required for meal preparation. The model also predicts how much time is needed to deliver a specific meal. Intelligence AI calculates all of that before the order is issued.
When an UberEATS product user places an order, it is sent to the particular restaurant for processing. The restaurant then needs to read and understand the request. Artificial Intelligence helps in here by decomposing orders and inputting them to restaurants IT systems.
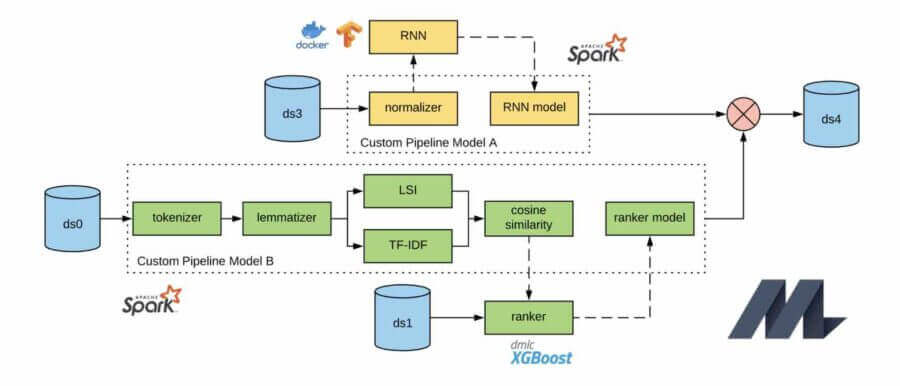
Figure 5: Michelangelo platform structure with specific data-driven tools involved in the processes (Source: Uber)
UberEats collects a lot of data within a single order delivery process. This data is collected, cleaned, and stored. It is then used to retrain models and deep learning neurons. There are more challenges for the use of AI though.
When the meal is close to being ready, and UberEats courier is dispatched to pick up the meal. Afterward, the delivery-partner needs to get to the venue, find a parking spot, collect the meal, then drive to the customer’s location.
Artificial Intelligence assists the deliver-partner through the whole process. It helps to bypass traffic, select parking spot, find the customer doors, and get back smoothly to the next pick up location.
Again the courier needs to use the navigation system to get to the venue, find parking, and walk to the customer’s door to complete the shipment.
Is it Machine Learning, Deep Learning, or both?
UberEats product management cycle leveraged gradient boosted decision tree regression models to predict this end-to-end delivery time.
Data points include information from the customer request (e.g., time of day, delivery location), historical features (e.g., average meal prep time for the specific time frame), and near-real-time calculated features (e.g., average meal prep time for the last one hour).
During the product management process, models are deployed across Uber’s data centers and are requested via a network by the UberEats microservices.
Final word
Artificial Intelligence already happened. Just like all the other groundbreaking technologies that have come before it, AI has begun to eat the software world. New Product Development already absorbed AI, and those companies that understand it can bring more value in a shorter time with lower costs.
Businesses that will not adopt this technology will soon be left in the dust by the competition to realize the tremendous profitability Artificial Intelligence brings to the table.