Data is the raw material that plays an important role in predictive analytics. It includes structured and unstructured data from various sources, such as customer data, transaction data, social media data, website traffic data, and more. The importance of data can be seen through various business operations and business processes. The data collected must be of high quality, relevant, and comprehensive to create accurate models.
What is Predictive Analytics?
Predictive analytics has become increasingly popular in recent years due to advances in technology, the availability of large amounts of data, and the growing need for data-driven decision-making in businesses and organizations.
Predictive analytics is a data-driven approach to forecasting future events or trends by analyzing past data patterns. It has become increasingly popular in recent years as a means of gaining insights into complex systems and making better decisions.
Predictive analytics is the use of statistical algorithms, machine learning techniques, and data mining methods to analyze historical data and make predictions about future events. Data plays a critical role in predictive analytics, as it forms the basis for creating models and predicting future outcomes.
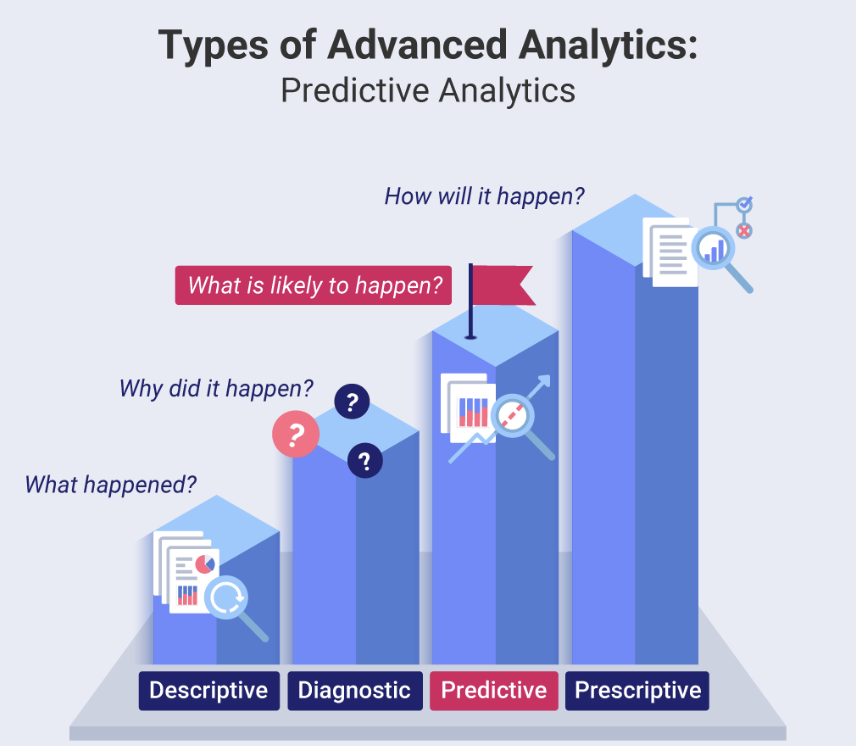
Steps to Use Data in Predictive Analysis
The process of predictive analytics typically involves several steps, including data collection, data preprocessing, model training, model evaluation, and deployment.
Data collection involves gathering data from various sources, such as databases, sensors, and social media platforms. Data preprocessing involves cleaning and transforming the data to prepare it for analysis. Data cleaning includes identifying and correcting errors, removing duplicates, filling in missing values, and standardizing data formats. Data transformation involves converting the data into a suitable format for analysis.
Once the data is prepared, the next step is to create a model. A model is a representation of a real-world system that uses data to make predictions about future outcomes. There are different types of models, including regression models, decision trees, neural networks, and others. Each model has its strengths and weaknesses, and the choice of model depends on the nature of the problem and the available data.
Model training involves selecting an appropriate algorithm and training it on historical data to create a predictive model. Model evaluation involves testing the model’s accuracy and making any necessary adjustments to improve its performance. Deployment involves integrating the predictive model into business processes and systems to make predictions and inform decision-making.
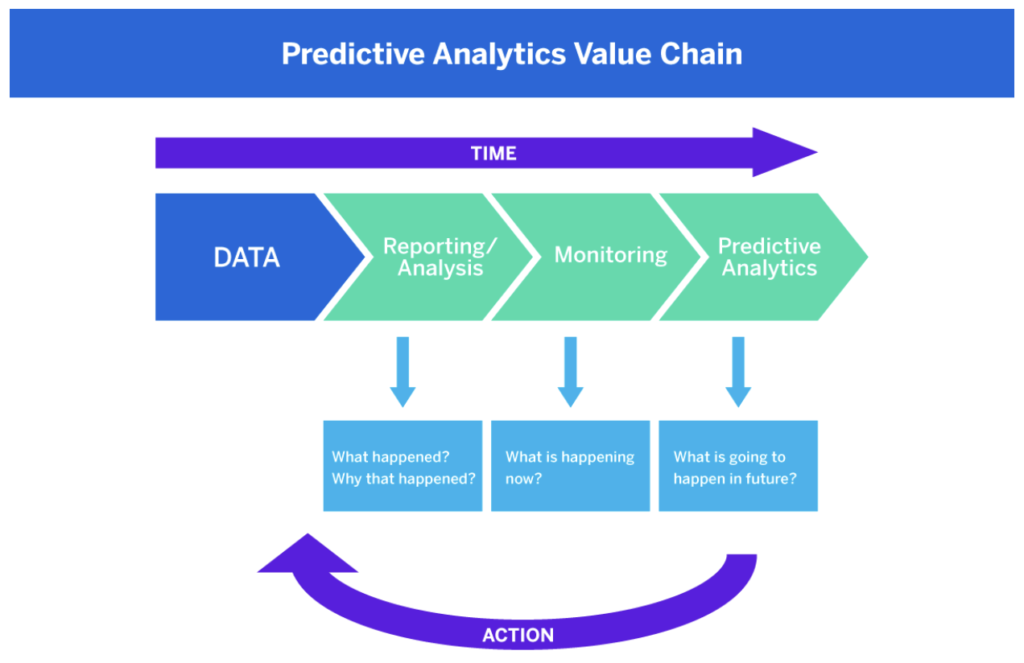
Benefits of Predictive Analysis
Predictive analysis has become an essential tool for businesses and organizations to promote business performance and make data-driven decisions. Here are some of the benefits of predictive analytics:
Improved Decision Making
Predictive analysis provides businesses with lots of information that can be used to make informed decisions. Predictive analysis allows businesses to identify patterns and trends in their data that can be used to make predictions about future events. This information can be used to improve decision-making processes and enable key strategic initiatives for the future.
Increased Efficiency
Predictive analysis can help businesses improve their operational efficiency by identifying areas where they can reduce expenses and improve productivity. For example, predictive analysis can be used to identify bottlenecks in a manufacturing process and suggest ways to optimize it or solve issues.
Better Customer Insights
Predictive analysis can be used to gain insights into customer behavior and preferences. This information can be used to develop targeted marketing campaigns, improve customer engagement, and develop better products and services.
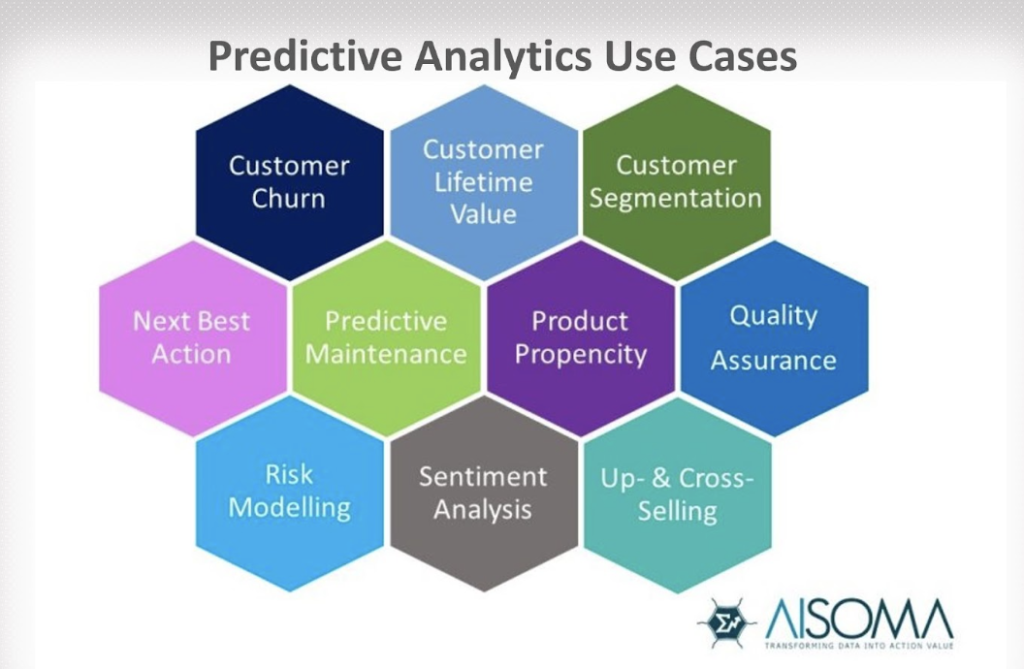
Examples of Using Data in Predictive Analysis
Predictive analytics has become increasingly popular across various industries and sectors due to its ability to help organizations make data-driven decisions. Here are some of the uses of predictive analytics across various industries:
Financial Services
In the financial services industry, predictive analytics can be used for credit scoring, fraud detection, and risk management. Banks and other financial institutions use predictive analytics to assess the creditworthiness of applicants and determine the likelihood of default. They also use it to detect fraudulent transactions and monitor risk.
Healthcare
Predictive analytics is used in healthcare to improve patient outcomes and reduce costs. Hospitals and healthcare providers use predictive analytics to identify patients who are at risk of developing certain diseases or complications. This allows healthcare providers to intervene early and prevent serious health issues from occurring.
Marketing
Predictive analytics can be used in marketing to analyze customer behavior or preferences and predict future behavior. Companies can use predictive analytics to create targeted marketing campaigns that are more likely to convert leads into customers. They can also use predictive analytics to forecast future sales and market changes and adjust their marketing strategies accordingly.
Manufacturing
Predictive analytics is used in manufacturing to optimize production processes and reduce downtime and costs. By analyzing data from sensors and machines, manufacturers can predict when equipment will likely fail and take preventative measures to avoid downtime. This helps to improve productivity and reduce maintenance costs.
Retail
In the retail industry, predictive analytics can be used to optimize inventory management and pricing strategies. Retailers can use predictive analytics to forecast supply and demand trends and ensure that they have the right amount of inventory on hand. They can also use predictive analytics to adjust pricing strategies based on customer behavior and market trends.
Conclusion
In conclusion, data plays a critical role in predictive analytics. It forms the basis for creating models and predicting future outcomes. However, the quality of the data is equally important, and data preparation, model training, and validation are essential to ensure accurate predictions. Predictive analytics has numerous applications across various industries, and its popularity is expected to grow as more data becomes available and as the tools and techniques for analysis continue to improve.